Modeling Irregularly Spaced Residual Series as a Continuous Stochastic Process
Abstract
Learning continuous-time stochastic dynamics is a fundamental and essential problem in modeling irregular time series, whose observations are irregular and sparse in both time and dimension. For a given system whose latent states and observed data are multivariate, it is generally impossible to derive a precise continuous-time stochastic process to describe the system behaviors. To solve the above problem, we apply Variational Bayesian method and propose a flexible continuous-time stochastic recurrent neural network named Variational Stochastic Differential Networks (VSDN), which embeds the complicated dynamics of the irregular time series by neural Stochastic Differential Equations (SDE). VSDNs capture the stochastic dependency among latent states and observations by deep neural networks. We also incorporate two differential Evidence Lower Bounds to efficiently train the models. Through comprehensive experiments, we show that VSDNs outperform state-of-the-art continuous-time deep learning models and achieve remarkable performance on prediction and interpolation tasks for irregular time series.
Keywords
- Neural Stochastic Differential Equations
- Stochastic recurrent neural network
- Irregular time series
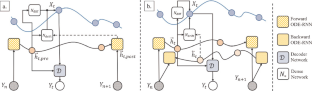
References
-
Archambeau, C., Opper, M., Shen, Y., Cornford, D., Shawe-taylor, J.S.: Variational inference for diffusion processes. In: Advances in Neural Information Processing Systems 20 (2008)
-
Burda, Y., Grosse, R., Salakhutdinov, R.: Importance weighted autoencoders. ArXiv arXiv:1509.00519 (2016)
-
Burgess, C.P., et al.: Understanding disentangling in \(\beta \)-VAE (2018)
-
Chen, T.Q., Rubanova, Y., Bettencourt, J., Duvenaud, D.K.: Neural ordinary differential equations. In: Advances in Neural Information Processing Systems vol. 31, pp. 6571–6583 (2018)
-
Chung, J., Kastner, K., Dinh, L., Goel, K., Courville, A.C., Bengio, Y.: A recurrent latent variable model for sequential data. In: Advances in Neural Information Processing Systems, vol. 28, pp. 2980–2988 (2015)
-
Cremer, C., Morris, Q., Duvenaud, D.: Reinterpreting importance-weighted autoencoders. In: International Conference on Learning Representations (ICLR) - Workshop Track (2017)
-
De Brouwer, E., Simm, J., Arany, A., Moreau, Y.: GRU-ODE-Bayes: continuous modeling of sporadically-observed time series. In: Advances in Neural Information Processing Systems, vol. 32, pp. 7379–7390 (2019)
-
Higgins, I., et al.: \(\beta \)-VAE: Learning basic visual concepts with a constrained variational framework. In: International Conference on Learning Representations (ICLR) (2017)
-
Ionescu, C., Papava, D., Olaru, V., Sminchisescu, C.: Human3.6m: large scale datasets and predictive methods for 3d human sensing in natural environments. IEEE Trans. Pattern Anal. Mach. Intell. 36(7), 1325–1339 (2014)
-
Kingma, D.P., Welling, M.: Auto-encoding variational bayes. In: International Conference on Learning Representations (ICLR) (2014)
-
Li, X., Wong, T.K.L., Chen, R.T.Q., Duvenaud, D.: Scalable gradients for stochastic differential equations. In: 23rd International Conference on Artificial Intelligence and Statistics, pp. 3870–3882 (August 2020)
-
Liu, Y., Xie, D., Wang, X.: Generalized boltzmann machine with deep neural structure. In: The 22nd International Conference on Artificial Intelligence and Statistics (AISTATS), vol. 89, pp. 926–934 (April 2019)
-
Martinez, J., Hossain, R., Romero, J., Little, J.J.: A simple yet effective baseline for 3d human pose estimation. In: IEEE/CVF International Conference on Computer Vision (ICCV) (2017)
-
Rainforth, T., et al.: Tighter variational bounds are not necessarily better. In: Proceedings of the 35th International Conference on Machine Learning, pp. 4274–4282 (2018)
-
Rubanova, Y., Chen, T.Q., Duvenaud, D.K.: Latent ordinary differential equations for irregularly-sampled time series. In: Advances in Neural Information Processing Systems, vol. 32, pp. 5321–5331 (2019)
-
Sussillo, D., Józefowicz, R., Abbott, L.F., Pandarinath, C.: LFADS - latent factor analysis via dynamical systems. ArXiv arXiv:1608.06315 (2016)
Acknowledgments
This work has been supported by NSF OIA 2040599, NSF OIA 2134840 and DOE 38456.
Author information
Authors and Affiliations
Editor information
Editors and Affiliations
Rights and permissions
Copyright information
© 2021 Springer Nature Switzerland AG
About this paper
Cite this paper
Liu, Y. et al. (2021). Continuous-Time Stochastic Differential Networks for Irregular Time Series Modeling. In: Mantoro, T., Lee, M., Ayu, M.A., Wong, K.W., Hidayanto, A.N. (eds) Neural Information Processing. ICONIP 2021. Communications in Computer and Information Science, vol 1516. Springer, Cham. https://doi.org/10.1007/978-3-030-92307-5_40
Download citation
- .RIS
- .ENW
- .BIB
-
DOI : https://doi.org/10.1007/978-3-030-92307-5_40
-
Published:
-
Publisher Name: Springer, Cham
-
Print ISBN: 978-3-030-92306-8
-
Online ISBN: 978-3-030-92307-5
-
eBook Packages: Computer Science Computer Science (R0)
Source: https://link.springer.com/chapter/10.1007/978-3-030-92307-5_40
0 Response to "Modeling Irregularly Spaced Residual Series as a Continuous Stochastic Process"
Post a Comment